Bayesian surplus production model with serial autocorrelation
DOI:
https://doi.org/10.47193/mafis.3212019061803Keywords:
Surplus production, serial autocorrelation, stock assessment, Bayesian estimate, Micropogonias furnieriAbstract
Presentation is made of a simple surplus production model called Surplus Production Model with Serial Autocorrelation (MPECAS in Spanish) since it considers as a unique assumption that the surplus production shows a serial correlation and has no explicit functional relation with biomass. Its application requires only an abundance index proportional to a given power of the actual mean abundance of the resource and the corresponding annual catches series. The estimate of the model parameters is presented within a Bayesian context using the SIR (Sampling Importance Resampling) algorithm. Simple risk criteria are proposed to estimate the Maximum Biologically Acceptable Catch (MBAC) and the risks associated to each hypothetical catch level considered. A simulation exercise was performed to assess the statistical capability of MPECAS to reproduce the information provided by a Schaefer operational surplus production model considered as an actual one. Finally, an application example with the white croaker (Micropogonias furnieri) is presented and the MBAC for 5 and 10% risk of biomass decline the year following the assessment year calculated with the Schaefer and MPECAS models are shown.
† Lic. Daniel R. Hernández passed away on january 25, 2019.
Downloads
References
Bernardo JM, Smith AFM. 2000. Bayesian Theory. Chichester: Wiley & Sons. 586 p.
Harvey AC. 1981. Time Series Models. Oxford: Philip Allan Publishers Limited. 229 p.
Hilborn R, Walters CJ. 1992. Quantitative Fisheries Stock Assessment. Choice, Dynamics and Uncertainty. Nueva York: Chapman & Hall. 570 p.
McAllister M, Kirchner C. 2002. Accounting for structural uncertainty to facilitate precautionary fishery management: illustration with namibian orange roughy. Bull Mar Sci. 70 (2): 499-540.
Punt E, Hilborn R. 1996. BIODYN. Biomass dynamic models. User’s manual. FAO Comput Inf Ser (Fish). 62 p.
Walters C, Ludwig D. 1994. Calculation of Bayes Posterior Probability Distributions for Key Population Parameters. Can J Fish Aquat Sci. 51: 713-722.
Zellner A. 1987. An Introduction to Bayesian Inference in Econometrics. Malabar: Robert E. Krieger Publishing Company. 431 p.
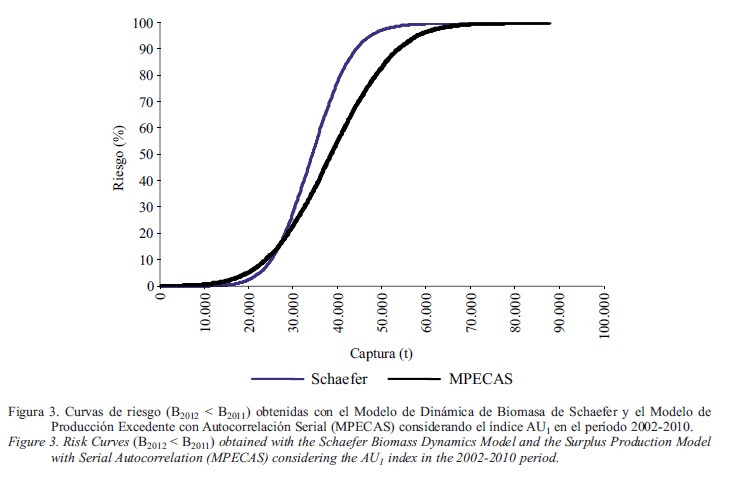
Downloads
Published
Issue
Section
License
Copyright (c) 2020 Daniel Hernández † , Julieta Rodríguez

This work is licensed under a Creative Commons Attribution-NonCommercial-ShareAlike 4.0 International License.
Authors of articles published in Marine and Fishery Sciences retain copyright on their articles, except for any third-party images and other materials added by Marine and Fishery Sciences, which are subject to copyright of their respective owners. Authors are therefore free to disseminate and re-publish their articles, subject to any requirements of third-party copyright owners and subject to the original publication being fully cited. Visitors may also download and forward articles subject to the citation requirements. The ability to copy, download, forward or otherwise distribute any materials is always subject to any copyright notices displayed. Copyright notices must be displayed prominently and may not be obliterated, deleted or hidden, totally or partially.
This journal offers authors an Open Access policy. Users are allowed to read, download, copy, distribute, print, search, or link to the full texts of the articles, or use them for any other legal purpose within the Creative Commons 4.0 license (BY-NC-SA), without asking prior permission from the publisher or the author. This is in accordance with the BOAI definition of Open Access.